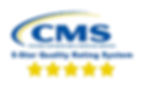
As health plans know, improving STAR ratings is an ongoing effort that requires a serious commitment of resources and technology
If done properly, however, organizations can benefit from more efficient processes and improved member experience/retention.
Improving STAR rating levels can also lead to coveted financial payouts from CMS. 🔝 🔝
Between ever-changing evaluation criteria across 40 STAR measures, weights, cut-points, utilization patterns, and member behavior, health plans need to continuously analyze incoming data and revise their STAR strategies accordingly.
Developing an MLOps platform can create an analytics framework that can predict the performance of STAR measures, identify priority measures and drive action steps, particularly for those measures that have the greatest impact on STAR scores and CMS reimbursement.
There are 4 areas where MLOps models can make a significant difference in moving the STAR needle:
1️⃣ Accurately predict and measure performance
ML models can be used to predict individual measure-level scores and cut-points across each of the 40 STAR measures, based on historical member data. After predictions are completed for measure-level scores, then weights can be applied to forecast a plan's overall STAR rating.
2️⃣ Identify priority measures
Once a health plan has its projected STAR scores, ML models can be used to predict which measures will drive the greatest overall STAR score improvement. This will ensure that time and resources are efficiently allocated.
3️⃣ Recommend member outreach
Now that priority STAR measures have been established, ML models can create propensity scores for members who are most likely to engage with outreach and those likely to close gaps in care related to STAR measures.
Further, ML algorithms, utilizing member profiles, can predict the types of outreaches that members are most receptive to, such as automated calls, emails, texts, digital health campaigns, etc.
4️⃣ Provide real-time inferences and insights to fine-tune measurement priorities and outreach strategies.
Once campaigns are live, outreach results can be analyzed to identify the most successful interventions and refine those that are underperforming. Retraining ML models with fresh data is key to continuous improvement which will enhance the accuracy of predictions, insights and alerts.
Developing a comprehensive MLOps platform will fundamentally change the way plans approach and resource their CMS STAR strategy (not to mention HEDIS, CAHPS and others). The ideal scenario is to match priority STAR measures with high-value members coupled with optimal outreach campaigns. ML may just be your secret weapon!
Interested to learn more? ➡️ Springer Nature
And don't forget to follow us!