ML vs. GAI: Transforming Healthcare and Life Sciences
- Diego Massara
- Aug 11, 2024
- 3 min read
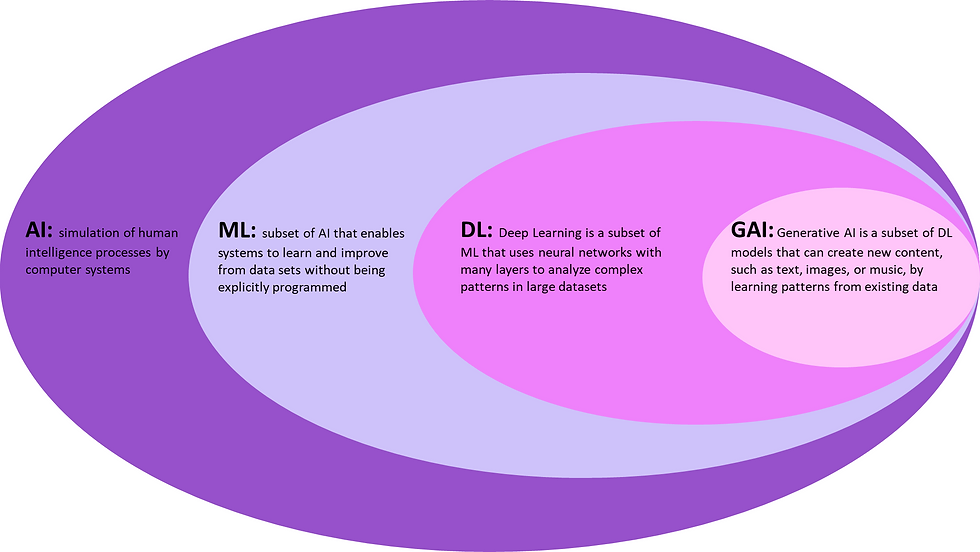
Understanding Machine Learning and Generative AI in Healthcare and Life Sciences 🧠
In the rapidly evolving world of healthcare and life sciences, two technological titans are reshaping the landscape: Machine Learning (ML) and Generative AI (GAI). While both are rooted in the principles of artificial intelligence, they serve distinct yet complementary roles in advancing medical research, patient care, and drug discovery. Let's dive into how these technologies are transforming the field and the unique contributions each brings to the table.
Machine Learning: The Analytical Maestro📊
Machine Learning has become a cornerstone of modern healthcare, offering a potent tool for data analysis and predictive modeling. At its core, ML involves algorithms that learn from historical data to make informed predictions and decisions. This capability is invaluable in a domain where data is abundant yet complex.
1️⃣ Diagnostics: ML models excel at analyzing medical images, such as X-rays and MRIs, to assist radiologists in diagnosing conditions with higher accuracy and speed.
2️⃣ Predictive Analytics: By sifting through vast datasets, ML can forecast patient outcomes, disease progression, and treatment efficacy, enabling proactive and personalized healthcare interventions.
3️⃣ Personalized Medicine: ML helps tailor treatments based on individual genetic profiles, offering personalized therapy plans that maximize efficacy and minimize side effects.
The strength of ML lies in its ability to enhance existing processes, making them more efficient and accurate. However, its focus is primarily on interpreting and analyzing data rather than creating new data.
Generative AI: The Creative Innovator 💡
Generative AI is the creative counterpart to ML. Generative AI encompasses models like Generative Adversarial Networks (GANs) and Transformers, designed to generate new data that mimics the properties of the training set. This capability opens up a world of possibilities in healthcare and life sciences.
1️⃣ Drug Discovery: One of the most promising applications of Generative AI is in drug discovery. By learning from existing chemical compounds, these models can propose novel molecular structures, accelerating the development of new drugs.
2️⃣ Synthetic Data Generation: Generative AI can create synthetic patient data, offering a valuable resource for training other AI models while preserving patient privacy.
3️⃣ Medical Imaging: It can enhance or generate medical images, providing richer datasets for training ML models or assisting in diagnostic processes.
Generative AI's focus is on innovation and creation, bringing forth new solutions and augmenting existing datasets with synthetic data, thus expanding the horizons of what is possible in medical research and treatment.
The Foundation: Data Lakehousing and Governance 🔑
The foundation upon which Machine Learning (ML) and Generative AI (GAI) applications are built is crucial for their success. Central to this foundation are robust data lakehousing and governance strategies. These elements ensure that data is not only stored efficiently but also managed and utilized effectively and responsibly to drive meaningful insights and innovations.
Implementing Generative AI (GAI) without a solid data lakehouse solution and data governance foundation can lead to business risks and even potential harm. Unlike traditional Machine Learning (ML), which relies on analyzing existing data to make predictions or optimize processes, GAI generates new content, designs, or processes. This capability, while powerful, can also amplify the consequences of poor data management and governance.
Equilibrium Point has over 20 years of expertise in big data, data architecture, data quality and governance for healthcare, pharma, and life sciences. We have a track record of supporting successful business transformations at scale, benefitting millions of patients through billions of annual data points. We live and breathe the data challenges in our industry, including data disparity and quality, disconnected vendors, and diverse product offerings. EQP can integrate all your data points from multiple sources and large systems into a single, accessible data lakehouse repository. We have built cutting-edge ML models over the last 6 years that enabled early detection of diseases such as diabetes and mental health disorders, improving patient outcomes and reducing hospital readmissions. For clinical trials our models have helped study sites by streamlining patient recruitment and retention, optimizing data management and analysis, and supporting risk-based monitoring.
Contact us now for a complimentary discovery meeting to unleash the potential of a data lakehouse, machine learning, and our new generative AI solutions in your organization!
Comments