Can digital health, backed by AI/ML, improve health outcomes and care delivery? A resounding, yes! ⬇️
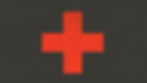
For decades. The healthcare industry has relied primarily on health insurance claims data for determining and measuring quality health metrics. However, flawed from the start, claims data are far from timely (3-6 months lag) and clinically incomplete.
Additionally, claims data are just a snapshot of a patient’s entirety of care, not a full and continuous view of a patient’s health journey.
The digital age of healthcare truly began with the mass transition to electronic health records (EHR) in 2010, driven by the Office of the National Coordinator for Health Information Technology. EHR’s are the foundation of the digital transformation in healthcare.
The challenge facing the industry now is how to develop the systems and technology that can accommodate a rapidly growing quantity and array of digital information sources and utilizing innovative technology, such as Artificial Intelligence (AI) and Machine Learning (ML), to harness the predictive power and insights to make further strides toward the Quadruple AIM in healthcare.
Although a tremendous leap forward, EHR’s alone are not providing the full picture of care. The advent and explosion of smartphones, wearable devices, fitness trackers, online patient assessments (HRA’s), consumer data and Social Determinants of Health (SDoH) data, have supplemented the breadth and depth of information available for analysis and quality measurement.How then to fully leverage the relatively new and powerful collection of data sources in a smart, responsible way?
1️⃣ Increase efficiency and timeliness in data collection efforts.
Create an infrastructure and data operations (DataOps) system that are flexible and adaptable to digital health data. This includes setting data quality standards led by a cross-functional Data Governance team.
2️⃣ Define and expand the end-to-end digital sources of clinical, patient and consumer information.
Patient reported data and SDoH data can provide critical pieces of information that provide insights into patient behavior and assessing quality of care. These include:
- Income and social status
- Employment and working conditions
- Education and literacy
- Physical environments
- Social supports and coping skills
- Access to health services
3️⃣ Integrate the DataOps platform with AI/ML technology
Data integrations to provide the gamut of healthcare players (patients, providers, payors, etc.), with real-time and personalized feedback, alerts, risk prediction, reminders and insights.
Taken together, these steps will create a digital environment that can more effectively and equitably care for the ‘whole person’ in the delivery and quality of health care.
Is your organization embracing the digital environment with DataOps and Machine Learning? 📝
Subscribe to our blog for more trending topics!